10 answers
Asked
723 views
what do I need to know as a data scientist?
I am an Msc student in data science and artificial intelligence. I had no previous background in programming hence I find it difficult to cope.
Login to comment
10 answers
Updated
Venkata Subbarao’s Answer
Hey Ridwan, As a data scientist you need to know about about data and it's impact on organization business. Simply, the relation between data and product/company. So, once you start doing research on a problem/task that depends on data belongs to the area of issue you will get lots of experience about data scientist role. However, to prove your findings technically you need to know programming knowledge like python, R..etc along with machine learning skill and some tools like Tubleau, pyspark tools etc. Overall, study about data just like study about other subjects. All the best!!
Updated
Benjamin’s Answer
Having a natural curiosity and the ability to critically analyze and approach problems is considered a driving factor in achieving success in data science, more so than simply knowing how to code. Possessing problem-solving skills allows you to properly evaluate the data returned during the data analysis process and derive meaningful insights from it.
While it is helpful to have a basic understanding of relevant mathematical concepts, it is not absolutely essential to have an extensive background in mathematics for a career in data science. Focusing on skills such as hypothesis testing and understanding the processes and methods involved would prove to be more beneficial in the long run.
To build a successful career in data science, it is important to develop a conceptual understanding of the key principles and methodologies used in the field, rather than becoming an expert in a specific coding language or mathematical concept. Learning commonly used tools and libraries, such as numpy, is useful, but having the exact knowledge of its internal workings may not be necessary.
To enhance your data science skills, it is crucial to focus on developing a strong foundation in the following areas:
1. Critical thinking and problem-solving: Cultivating the ability to analyze and interpret data, assess assumptions and biases, and identify patterns and trends.
2. Communication and data visualization: Effectively conveying your findings and insights to non-technical audiences through the use of data visualization tools and clear, concise language.
3. Domain knowledge: Understanding the context of the specific industry or field in which you are working, allowing you to make informed decisions based on existing knowledge.
4. Statistical concepts: Familiarizing yourself with key statistical concepts such as probability, sampling techniques, and hypothesis testing, used in data analysis and modeling.
5. Programming languages and tools: Acquiring a basic understanding of popular programming languages (such as Python or R) and tools used in data science to manipulate, clean, and analyze data efficiently.
In conclusion, having a natural curiosity and problem-solving mindset, together with a solid understanding of the core concepts and methodologies mentioned above, is an essential and comprehensive approach towards a successful career in data science. Proficiency in coding or an extensive background in math, while advantageous, is not a definitive requirement for success in this field.
While it is helpful to have a basic understanding of relevant mathematical concepts, it is not absolutely essential to have an extensive background in mathematics for a career in data science. Focusing on skills such as hypothesis testing and understanding the processes and methods involved would prove to be more beneficial in the long run.
To build a successful career in data science, it is important to develop a conceptual understanding of the key principles and methodologies used in the field, rather than becoming an expert in a specific coding language or mathematical concept. Learning commonly used tools and libraries, such as numpy, is useful, but having the exact knowledge of its internal workings may not be necessary.
To enhance your data science skills, it is crucial to focus on developing a strong foundation in the following areas:
1. Critical thinking and problem-solving: Cultivating the ability to analyze and interpret data, assess assumptions and biases, and identify patterns and trends.
2. Communication and data visualization: Effectively conveying your findings and insights to non-technical audiences through the use of data visualization tools and clear, concise language.
3. Domain knowledge: Understanding the context of the specific industry or field in which you are working, allowing you to make informed decisions based on existing knowledge.
4. Statistical concepts: Familiarizing yourself with key statistical concepts such as probability, sampling techniques, and hypothesis testing, used in data analysis and modeling.
5. Programming languages and tools: Acquiring a basic understanding of popular programming languages (such as Python or R) and tools used in data science to manipulate, clean, and analyze data efficiently.
In conclusion, having a natural curiosity and problem-solving mindset, together with a solid understanding of the core concepts and methodologies mentioned above, is an essential and comprehensive approach towards a successful career in data science. Proficiency in coding or an extensive background in math, while advantageous, is not a definitive requirement for success in this field.
Updated
Jacob’s Answer
For me, I have found competency-based master's programs to be helpful so the student can slow down when they are struggling to keep up with the rest of the class who have deeper backgrounds than I do in programming.
Updated
Rihem’s Answer
Hello Ridwan !
As a data scientist, you need to be more than a data analyst; you must be a data sorcerer, conjuring insights from the depths of data's mystique. Here's a unique perspective on what you need to know:
Data Alchemy: Master the art of transforming raw data into gold, with skills in data cleaning, preprocessing, and feature engineering. Your magic lies in turning messy, raw data into something valuable.
Statistical Wizardry: Possess a deep understanding of statistics and probability, casting spells to reveal patterns, correlations, and anomalies in your data.
Machine Learning Sorcery: Embrace the arcane world of machine learning, including both supervised and unsupervised techniques. Your power comes from understanding algorithms, model selection, and hyperparameter tuning.
Programming Enchantment: Be fluent in multiple programming languages, particularly Python and R, and wield the power to script and automate data processes.
Data Visualization Artistry: Create compelling data visualizations to communicate your findings with clarity and impact. Turn numbers into art that tells a story.
Big Data Conjuring: Command big data tools like Hadoop, Spark, and distributed computing platforms to handle vast datasets.
Domain Knowledge Sorcery: Understand the specific domain you're working in; your spells work best when you know the context and nuances of the data.
A/B Testing Wizardry: Master the art of designing and analyzing A/B tests to validate hypotheses and guide decision-making.
Storytelling Magic: Craft enchanting narratives from your data insights. Use storytelling to influence and guide decision-makers.
Ethical Enchantment: Always work ethically with data, respecting privacy and ensuring your spells don't cause harm.
Continuous Learning Grimoire: Your magic must continually evolve; the world of data is ever-changing. Stay up-to-date with new techniques, tools, and technologies.
Data Engineering Conjuration: Collaborate with data engineers to build and maintain data pipelines that keep your spells well-fed with high-quality data.
Ensemble Sorcery: Combine different models and techniques to create more robust and accurate predictions. Your predictive power grows with your ensemble spells.
Open Source Potions: Utilize open-source libraries and tools to brew your data potions. Communities like GitHub and Kaggle are treasure troves.
Data Story Alchemy: Craft data-driven stories that resonate with your audience, mixing data, context, and insights into an enchanting narrative.
Data Privacy Spells: Be versed in data privacy regulations and spell out protective enchantments to safeguard sensitive information.
Humble Apprentice: Understand that no one knows it all; be humble and open to learning from others, as the realm of data is vast and diverse.
Communication Elixir: Perfect the elixir of clear and effective communication. Your spells are useless if you can't convey their magic to non-technical audiences.
Becoming a proficient data scientist involves mastering a unique blend of technical skills, domain knowledge, and storytelling abilities. You are the modern-day alchemist, turning data into insights and creating magical solutions that impact businesses and society.
As a data scientist, you need to be more than a data analyst; you must be a data sorcerer, conjuring insights from the depths of data's mystique. Here's a unique perspective on what you need to know:
Data Alchemy: Master the art of transforming raw data into gold, with skills in data cleaning, preprocessing, and feature engineering. Your magic lies in turning messy, raw data into something valuable.
Statistical Wizardry: Possess a deep understanding of statistics and probability, casting spells to reveal patterns, correlations, and anomalies in your data.
Machine Learning Sorcery: Embrace the arcane world of machine learning, including both supervised and unsupervised techniques. Your power comes from understanding algorithms, model selection, and hyperparameter tuning.
Programming Enchantment: Be fluent in multiple programming languages, particularly Python and R, and wield the power to script and automate data processes.
Data Visualization Artistry: Create compelling data visualizations to communicate your findings with clarity and impact. Turn numbers into art that tells a story.
Big Data Conjuring: Command big data tools like Hadoop, Spark, and distributed computing platforms to handle vast datasets.
Domain Knowledge Sorcery: Understand the specific domain you're working in; your spells work best when you know the context and nuances of the data.
A/B Testing Wizardry: Master the art of designing and analyzing A/B tests to validate hypotheses and guide decision-making.
Storytelling Magic: Craft enchanting narratives from your data insights. Use storytelling to influence and guide decision-makers.
Ethical Enchantment: Always work ethically with data, respecting privacy and ensuring your spells don't cause harm.
Continuous Learning Grimoire: Your magic must continually evolve; the world of data is ever-changing. Stay up-to-date with new techniques, tools, and technologies.
Data Engineering Conjuration: Collaborate with data engineers to build and maintain data pipelines that keep your spells well-fed with high-quality data.
Ensemble Sorcery: Combine different models and techniques to create more robust and accurate predictions. Your predictive power grows with your ensemble spells.
Open Source Potions: Utilize open-source libraries and tools to brew your data potions. Communities like GitHub and Kaggle are treasure troves.
Data Story Alchemy: Craft data-driven stories that resonate with your audience, mixing data, context, and insights into an enchanting narrative.
Data Privacy Spells: Be versed in data privacy regulations and spell out protective enchantments to safeguard sensitive information.
Humble Apprentice: Understand that no one knows it all; be humble and open to learning from others, as the realm of data is vast and diverse.
Communication Elixir: Perfect the elixir of clear and effective communication. Your spells are useless if you can't convey their magic to non-technical audiences.
Becoming a proficient data scientist involves mastering a unique blend of technical skills, domain knowledge, and storytelling abilities. You are the modern-day alchemist, turning data into insights and creating magical solutions that impact businesses and society.
Updated
Jennifer’s Answer
Hello there! Embarking on the journey to become a data analyst is an exciting one, full of opportunities to learn and grow. A good starting point would be to familiarize yourself with Excel and Google Sheets. Once you've got a good handle on these, it's time to dive into the world of code writing. Languages like SQL and Python will become your new best friends.
But it's not just about numbers and codes. A successful data analyst also knows how to weave data into compelling stories. Luckily, there are fantastic software programs, such as Tableau, that can assist you in mastering this art of data storytelling.
Don't worry about the cost of learning. The internet is brimming with free training resources. Additionally, you can find helpful books like "SQL for Dummies" on Amazon to kickstart your learning journey.
Remember, teamwork makes the dream work! Collaborating with fellow data analysts can greatly enhance data accuracy and the quality of the final product. As for me, I currently work with telecommunications data.
Earning certifications can give you a significant boost on this journey. So, gear up and get ready to embrace the fascinating world of data analysis!
But it's not just about numbers and codes. A successful data analyst also knows how to weave data into compelling stories. Luckily, there are fantastic software programs, such as Tableau, that can assist you in mastering this art of data storytelling.
Don't worry about the cost of learning. The internet is brimming with free training resources. Additionally, you can find helpful books like "SQL for Dummies" on Amazon to kickstart your learning journey.
Remember, teamwork makes the dream work! Collaborating with fellow data analysts can greatly enhance data accuracy and the quality of the final product. As for me, I currently work with telecommunications data.
Earning certifications can give you a significant boost on this journey. So, gear up and get ready to embrace the fascinating world of data analysis!
Updated
John’s Answer
Id be tempted to take an industry credential/badge in Data Science to round out what you need to know - while also getting an industry badge at the same time.
IBM Skills Build is totally free - and has great, concise courses on Data Science - ill pop the link in the Next Steps
IBM Skills Build Data Science can be found here - https://www.ibm.com/academic/topic/data-science
IBM Skills Build is totally free - and has great, concise courses on Data Science - ill pop the link in the Next Steps
John recommends the following next steps:
Updated
DAve’s Answer
Begin by checking out an online learning platform, such as Datacamp. This platform will offer various roles that you can delve into, along with related learning resources. Yes, there might be a cost involved, but you can start with a small investment. Dedicate a solid month to really concentrate on it.
Updated
Preethu Pallavi’s Answer
There can be 2 types of data science jobs you might majorly come across:
1.Core Products Related
This can include building realtime data science solutions , deploying them and maintaining and scaling such solutions for your customer base.
You will need to know coding, and some part on how to deploy your Solutions , though there are many tools that can ease this process for you knowing to code can be of great use.
2.Analytical Business or Finance related.
In such job domains you don't need very good coding skills , you will need to be able to use any of your learnings and apply it to answer critical business questions on how well a product is doing?some predictions on you customer purchases or any such questions you leadership or Product Managers might have in order to take proper decisions.You will need knowledge of some visualization tools like Tableau/Mode/Power BI which can be used to showcase you results.But even in this profile you will need basic coding skills of writing standalone scripts ,coding some of the DS/ML models, some basics of data cleaning and good knowledge of SQL to get the data needed.
To answer you question :
You will need some knowledge of coding for Data Science but you will not be expected to learn all the Data Structures/Algorithms you might see on Leetcode or such platforms, those are fro core Software Developers.
I would suggest learning basics of Python (2-3 weeks),then implement a few ML models basic ones(1 week ) you will be able to find many online datasets on which you can do your learning.In a month you will have your basics set , from there on you can improve your coding skills gradually.
Coding might be needed for data science, but we also have many tools that can do that for you but knowing to code would be a plus.But most of all
it's important to develop critical thinking, problem solving skills as a data scientist once you know a problem you want to solve with data , finding a solution to it is not that difficult with basics of programming.
Learn the basics of Programming
Implement a few projects , simple classification or prediction model on any data set can do.
After you implementation , what are the key takeaways you notice , what are some decisions/learnings about the data from your solution .Answer this will make you a better data scientist
1.Core Products Related
This can include building realtime data science solutions , deploying them and maintaining and scaling such solutions for your customer base.
You will need to know coding, and some part on how to deploy your Solutions , though there are many tools that can ease this process for you knowing to code can be of great use.
2.Analytical Business or Finance related.
In such job domains you don't need very good coding skills , you will need to be able to use any of your learnings and apply it to answer critical business questions on how well a product is doing?some predictions on you customer purchases or any such questions you leadership or Product Managers might have in order to take proper decisions.You will need knowledge of some visualization tools like Tableau/Mode/Power BI which can be used to showcase you results.But even in this profile you will need basic coding skills of writing standalone scripts ,coding some of the DS/ML models, some basics of data cleaning and good knowledge of SQL to get the data needed.
To answer you question :
You will need some knowledge of coding for Data Science but you will not be expected to learn all the Data Structures/Algorithms you might see on Leetcode or such platforms, those are fro core Software Developers.
I would suggest learning basics of Python (2-3 weeks),then implement a few ML models basic ones(1 week ) you will be able to find many online datasets on which you can do your learning.In a month you will have your basics set , from there on you can improve your coding skills gradually.
Coding might be needed for data science, but we also have many tools that can do that for you but knowing to code would be a plus.But most of all
it's important to develop critical thinking, problem solving skills as a data scientist once you know a problem you want to solve with data , finding a solution to it is not that difficult with basics of programming.
Preethu Pallavi recommends the following next steps:
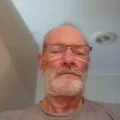
James Constantine Frangos
Consultant Dietitian & Software Developer since 1972 => Nutrition Education => Health & Longevity => Self-Actualization.
6176
Answers
Gold Coast, Queensland, Australia
Updated
James Constantine’s Answer
Hey there, Ridwan!
In your exciting journey as a data scientist, there are some key concepts and skills you'll need to get a good grip on. These are the ones that are currently in demand, based on the latest industry trends and best practices:
Data Programming and Crunching
1. Mastering programming languages: Python, R, and SQL are the top dogs in the programming world of data science. Get comfortable with at least one of them and practice writing code to crack data-related puzzles.
2. Tidying up data: Get the hang of cleaning, preprocessing, and tweaking data using handy libraries and tools like Pandas, NumPy, and Matplotlib.
3. Painting with data: Learn to craft effective visualizations using tools like Tableau, Power BI, or D3.js to share your findings with others.
4. Diving into machine learning and deep learning: Explore machine learning algorithms and their uses in data science, including supervised and unsupervised learning, regression, classification, clustering, and neural networks.
5. Tackling Big Data: Get acquainted with big data tools like Hadoop, Spark, and NoSQL databases for handling massive data processing and analysis.
Data Analysis and Decoding
1. Statistics savvy: Get a handle on statistical concepts like hypothesis testing, confidence intervals, and regression analysis to read data results accurately.
2. Mining data and choosing features: Learn to pull out meaningful nuggets from large datasets and pick out relevant features for modeling.
3. Spinning data tales: Hone your ability to explain complex data findings to non-techy folks through engaging stories and visuals.
4. Industry know-how: Get to know a specific industry or domain to apply data science concepts to real-life problems and understand the business backdrop.
Teamwork and Communication
1. Communication chops: Build strong communication skills to present complex data findings to all types of people, tech-savvy or not.
2. Team player: Learn to work well in teams, talk with different people, and manage projects to deliver data-driven solutions.
3. Industry know-how: Get to know a specific industry or domain to apply data science concepts to real-life problems and understand the business backdrop.
Ethical Thinking
1. Privacy and security: Understand the ethical side of collecting, storing, and processing sensitive data, and why data privacy and security matter.
2. Fairness and bias: Learn to spot and lessen biases in data and models, and ensure fairness in decision-making processes.
3. Clarity and explanation: Hone your ability to explain complex data findings and models to different people, and ensure clarity in data-driven decision-making processes.
Professional Growth
1. Keeping up with the Joneses: Join conferences, workshops, and online forums to stay in the loop with the latest happenings in data science.
2. Networking: Grow a professional network of buddies, mentors, and industry experts to learn from their experiences and stay in touch with the data science community.
3. Lifelong learning: Adopt a growth mindset and keep learning new skills and technologies to stay relevant in the fast-paced world of data science.
References:
1. "Data Science Handbook" by Jake VanderPlas (2018) - Your go-to guide for everything data science, from programming to data analysis, to machine learning.
2. "Python Data Science Handbook" by Jake VanderPlas (2017) - A practical guide to navigating data science with Python, covering data cleaning, visualization, and machine learning.
3. "Hands-On Machine Learning with Scikit-Learn, Keras, and TensorFlow" by Aurelien Geron (2017) - A hands-on guide to machine learning with Python, covering supervised and unsupervised learning, and deep learning.
Being a data scientist means having a solid foundation in programming, data analysis, and machine learning, as well as the knack for explaining complex insights to others. Plus, keeping up with industry trends and best practices, and constantly learning new skills and technologies, are key to thriving in this ever-changing field.
In your exciting journey as a data scientist, there are some key concepts and skills you'll need to get a good grip on. These are the ones that are currently in demand, based on the latest industry trends and best practices:
Data Programming and Crunching
1. Mastering programming languages: Python, R, and SQL are the top dogs in the programming world of data science. Get comfortable with at least one of them and practice writing code to crack data-related puzzles.
2. Tidying up data: Get the hang of cleaning, preprocessing, and tweaking data using handy libraries and tools like Pandas, NumPy, and Matplotlib.
3. Painting with data: Learn to craft effective visualizations using tools like Tableau, Power BI, or D3.js to share your findings with others.
4. Diving into machine learning and deep learning: Explore machine learning algorithms and their uses in data science, including supervised and unsupervised learning, regression, classification, clustering, and neural networks.
5. Tackling Big Data: Get acquainted with big data tools like Hadoop, Spark, and NoSQL databases for handling massive data processing and analysis.
Data Analysis and Decoding
1. Statistics savvy: Get a handle on statistical concepts like hypothesis testing, confidence intervals, and regression analysis to read data results accurately.
2. Mining data and choosing features: Learn to pull out meaningful nuggets from large datasets and pick out relevant features for modeling.
3. Spinning data tales: Hone your ability to explain complex data findings to non-techy folks through engaging stories and visuals.
4. Industry know-how: Get to know a specific industry or domain to apply data science concepts to real-life problems and understand the business backdrop.
Teamwork and Communication
1. Communication chops: Build strong communication skills to present complex data findings to all types of people, tech-savvy or not.
2. Team player: Learn to work well in teams, talk with different people, and manage projects to deliver data-driven solutions.
3. Industry know-how: Get to know a specific industry or domain to apply data science concepts to real-life problems and understand the business backdrop.
Ethical Thinking
1. Privacy and security: Understand the ethical side of collecting, storing, and processing sensitive data, and why data privacy and security matter.
2. Fairness and bias: Learn to spot and lessen biases in data and models, and ensure fairness in decision-making processes.
3. Clarity and explanation: Hone your ability to explain complex data findings and models to different people, and ensure clarity in data-driven decision-making processes.
Professional Growth
1. Keeping up with the Joneses: Join conferences, workshops, and online forums to stay in the loop with the latest happenings in data science.
2. Networking: Grow a professional network of buddies, mentors, and industry experts to learn from their experiences and stay in touch with the data science community.
3. Lifelong learning: Adopt a growth mindset and keep learning new skills and technologies to stay relevant in the fast-paced world of data science.
References:
1. "Data Science Handbook" by Jake VanderPlas (2018) - Your go-to guide for everything data science, from programming to data analysis, to machine learning.
2. "Python Data Science Handbook" by Jake VanderPlas (2017) - A practical guide to navigating data science with Python, covering data cleaning, visualization, and machine learning.
3. "Hands-On Machine Learning with Scikit-Learn, Keras, and TensorFlow" by Aurelien Geron (2017) - A hands-on guide to machine learning with Python, covering supervised and unsupervised learning, and deep learning.
Being a data scientist means having a solid foundation in programming, data analysis, and machine learning, as well as the knack for explaining complex insights to others. Plus, keeping up with industry trends and best practices, and constantly learning new skills and technologies, are key to thriving in this ever-changing field.
Updated
Karthik’s Answer
1. Dive into the world of statistics & Grasp the fundamentals of machine learning.
2. Read ace the data science interview book & practice relevant interview questions on strata scratch for companied you're interested in
3. Identify real-world business scenarios where data science comes into play.
4. Master the art of data storytelling and visualization.
5. Understand and apply KPIs or metrics pertinent to your target industry or company.
2. Read ace the data science interview book & practice relevant interview questions on strata scratch for companied you're interested in
3. Identify real-world business scenarios where data science comes into play.
4. Master the art of data storytelling and visualization.
5. Understand and apply KPIs or metrics pertinent to your target industry or company.