9 answers
Asked
829 views
How do you pursue a career in data science?
I am majoring in computer science. What kind of projects could you build?
Login to comment
9 answers
Updated
David’s Answer
To pursue a career in data science, leverage your background in computer science to gain a solid foundation in programming, algorithms, and data structures. Familiarize yourself with key concepts in mathematics and statistics, including linear algebra, probability, and inferential statistics, as these form the basis for data analysis and modeling. Additionally, learn about data manipulation and analysis tools and libraries such as Python's pandas, NumPy, and scikit-learn, as well as programming languages commonly used in data science like R and SQL. Consider taking online courses or enrolling in university courses that cover data science topics, and actively participate in data-related projects or competitions to gain practical experience and build a portfolio.
As a computer science major, you have the opportunity to work on a wide range of data science projects that showcase your technical skills and creativity. For example, you could develop a predictive model to forecast stock prices or analyze sentiment from social media data using natural language processing techniques. You could also explore machine learning algorithms to classify images, detect anomalies in network traffic, or recommend products based on user preferences. Another option is to work on data visualization projects, creating interactive dashboards or visualizations to communicate insights from complex datasets effectively. Ultimately, the projects you choose to undertake should align with your interests and career goals in data science, allowing you to demonstrate your proficiency in data analysis, programming, and problem-solving.
As a computer science major, you have the opportunity to work on a wide range of data science projects that showcase your technical skills and creativity. For example, you could develop a predictive model to forecast stock prices or analyze sentiment from social media data using natural language processing techniques. You could also explore machine learning algorithms to classify images, detect anomalies in network traffic, or recommend products based on user preferences. Another option is to work on data visualization projects, creating interactive dashboards or visualizations to communicate insights from complex datasets effectively. Ultimately, the projects you choose to undertake should align with your interests and career goals in data science, allowing you to demonstrate your proficiency in data analysis, programming, and problem-solving.
Updated
Giacomo’s Answer
You need basis of computer science
After you can enroll in an university in presence of data science (better)
Or an online university. Prestigeous university are more appreciated . Example MIT
After you can enroll in an university in presence of data science (better)
Or an online university. Prestigeous university are more appreciated . Example MIT
Updated
Patrick’s Answer
Melissa, to kickstart a career in data science, particularly with your computer science background, it's crucial to establish a solid base in key skills. These include programming languages like Python, R, and SQL, statistical analysis, machine learning algorithms, and data handling methods. To bolster your academic learning, think about taking online classes, workshops, or earning certifications in data science and analytics.
Immersing yourself in data science projects is a great way to gain practical experience and showcase your abilities. As a computer science student, you can create projects that utilize your programming skills to gather, cleanse, examine, and visualize data. For example, you could develop projects like social media sentiment analysis, sales prediction models, recommendation systems for online shopping platforms, or image recognition apps using machine learning algorithms.
Such projects not only exhibit your technical skills but also let you delve into various data science sectors and customize your portfolio to align with your career goals and dreams. Additionally, think about joining hackathons, internships, or research opportunities to further polish your skills and gain hands-on experience in real-world data science situations. By actively engaging in projects and seeking learning opportunities, you can efficiently gear up for a thriving career in data science.
Immersing yourself in data science projects is a great way to gain practical experience and showcase your abilities. As a computer science student, you can create projects that utilize your programming skills to gather, cleanse, examine, and visualize data. For example, you could develop projects like social media sentiment analysis, sales prediction models, recommendation systems for online shopping platforms, or image recognition apps using machine learning algorithms.
Such projects not only exhibit your technical skills but also let you delve into various data science sectors and customize your portfolio to align with your career goals and dreams. Additionally, think about joining hackathons, internships, or research opportunities to further polish your skills and gain hands-on experience in real-world data science situations. By actively engaging in projects and seeking learning opportunities, you can efficiently gear up for a thriving career in data science.
Updated
Iqra’s Answer
Pursuing a career in data science involves acquiring a blend of technical skills, practical experience, and staying updated with industry trends. Here’s a comprehensive guide covering the various aspects you mentioned:
1. Python Coding
Why Python?: Python is a versatile and widely-used programming language in data science due to its simplicity and the extensive range of libraries available for data manipulation, analysis, and visualization.
Key Libraries: Learn libraries such as NumPy, Pandas, Matplotlib, Seaborn, and SciPy for data manipulation and visualization. For machine learning, familiarize yourself with Scikit-learn, TensorFlow, and PyTorch.
Practice: Work on coding exercises, participate in coding challenges on platforms like LeetCode, HackerRank, and Kaggle.
2. Databases
SQL: Master SQL for querying relational databases. Understand the basics of database design and normalization.
NoSQL: Learn about NoSQL databases like MongoDB, which are useful for handling unstructured data.
Data Warehousing: Gain knowledge of data warehousing solutions like Amazon Redshift, Google BigQuery, or Snowflake.
3. Machine Learning
Fundamentals: Study the basics of machine learning algorithms such as linear regression, decision trees, clustering, and neural networks.
Practical Application: Work on projects that involve building and deploying machine learning models. Use platforms like Kaggle to find datasets and participate in competitions.
Tools: Get comfortable with machine learning frameworks like Scikit-learn, TensorFlow, and PyTorch.
4. Following Data Science Influencers
Social Media and Blogs: Follow influential data scientists on Twitter, LinkedIn, and Medium. Some notable names include Andrew Ng, Cassie Kozyrkov, and Hilary Mason.
Webinars and Conferences: Attend webinars, workshops, and conferences to stay updated with the latest trends and network with professionals in the field.
5. Deep Learning
Advanced Techniques: Dive into advanced topics like convolutional neural networks (CNNs), recurrent neural networks (RNNs), and generative adversarial networks (GANs).
Courses and Resources: Take online courses from platforms like Coursera, edX, and Udacity. Andrew Ng’s deep learning specialization on Coursera is highly recommended.
Projects: Implement deep learning models on real-world datasets to gain hands-on experience.
6. ML System Design
Architecture: Learn about designing scalable and efficient machine learning systems. Understand the end-to-end process from data collection to model deployment.
Best Practices: Study best practices for model versioning, monitoring, and maintenance.
Tools: Familiarize yourself with tools like MLflow, Kubeflow, and TensorFlow Extended (TFX) for managing the machine learning lifecycle.
7. Large Language Models (LLMs)
Understanding LLMs: Learn about the architecture and functioning of large language models like GPT-3, BERT, and their applications in natural language processing (NLP).
Applications: Explore how LLMs can be used for tasks such as text generation, translation, summarization, and sentiment analysis.
Hands-On: Experiment with pre-trained models using libraries like Hug
1. Python Coding
Why Python?: Python is a versatile and widely-used programming language in data science due to its simplicity and the extensive range of libraries available for data manipulation, analysis, and visualization.
Key Libraries: Learn libraries such as NumPy, Pandas, Matplotlib, Seaborn, and SciPy for data manipulation and visualization. For machine learning, familiarize yourself with Scikit-learn, TensorFlow, and PyTorch.
Practice: Work on coding exercises, participate in coding challenges on platforms like LeetCode, HackerRank, and Kaggle.
2. Databases
SQL: Master SQL for querying relational databases. Understand the basics of database design and normalization.
NoSQL: Learn about NoSQL databases like MongoDB, which are useful for handling unstructured data.
Data Warehousing: Gain knowledge of data warehousing solutions like Amazon Redshift, Google BigQuery, or Snowflake.
3. Machine Learning
Fundamentals: Study the basics of machine learning algorithms such as linear regression, decision trees, clustering, and neural networks.
Practical Application: Work on projects that involve building and deploying machine learning models. Use platforms like Kaggle to find datasets and participate in competitions.
Tools: Get comfortable with machine learning frameworks like Scikit-learn, TensorFlow, and PyTorch.
4. Following Data Science Influencers
Social Media and Blogs: Follow influential data scientists on Twitter, LinkedIn, and Medium. Some notable names include Andrew Ng, Cassie Kozyrkov, and Hilary Mason.
Webinars and Conferences: Attend webinars, workshops, and conferences to stay updated with the latest trends and network with professionals in the field.
5. Deep Learning
Advanced Techniques: Dive into advanced topics like convolutional neural networks (CNNs), recurrent neural networks (RNNs), and generative adversarial networks (GANs).
Courses and Resources: Take online courses from platforms like Coursera, edX, and Udacity. Andrew Ng’s deep learning specialization on Coursera is highly recommended.
Projects: Implement deep learning models on real-world datasets to gain hands-on experience.
6. ML System Design
Architecture: Learn about designing scalable and efficient machine learning systems. Understand the end-to-end process from data collection to model deployment.
Best Practices: Study best practices for model versioning, monitoring, and maintenance.
Tools: Familiarize yourself with tools like MLflow, Kubeflow, and TensorFlow Extended (TFX) for managing the machine learning lifecycle.
7. Large Language Models (LLMs)
Understanding LLMs: Learn about the architecture and functioning of large language models like GPT-3, BERT, and their applications in natural language processing (NLP).
Applications: Explore how LLMs can be used for tasks such as text generation, translation, summarization, and sentiment analysis.
Hands-On: Experiment with pre-trained models using libraries like Hug
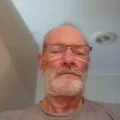
James Constantine Frangos
Consultant Dietitian & Software Developer since 1972 => Nutrition Education => Health & Longevity => Self-Actualization.
6084
Answers
Gold Coast, Queensland, Australia
Updated
James Constantine’s Answer
Dear Melissa,
ME:-
If for instance you have nutrition and dietetics and computing, using data you can make software that educates people about what the Standard Reference database contains. Levels of nutrients that can ameliorate sicknesses. An example is zinc supporting immune function.
THE AI:-
Charting a Path Towards a Data Science Career
Embarking on a data science career, particularly if you have a computer science background, involves several steps to boost your skills and improve your chances of success. Here's a detailed roadmap to navigate your way into a data science career:
1. Education and Skill Enhancement:
Pursue Relevant Education: Start by obtaining a degree in a related field such as computer science, statistics, mathematics, or data science. These areas form the bedrock of a data science career.
Enroll in Specialized Courses and Certifications: Consider signing up for specialized data science courses or earning certifications. Online platforms like Coursera, edX, and Udacity offer a variety of data science courses.
Hone Programming Skills: Mastery of programming languages like Python, R, SQL, and Java is vital for data science roles. Regular coding practice will solidify your skills.
Understand Statistics: Grasping statistical concepts and techniques is key to effectively analyzing and interpreting data.
2. Accumulate Practical Experience:
Seek Internships and Projects: Pursue internships or projects that enable you to use your knowledge in real-life situations. Practical experience will not only augment your skills but also make you more appealing to potential employers.
Join Kaggle Competitions: Engaging in Kaggle competitions can offer valuable experience in tackling intricate data problems and working with actual datasets.
3. Construct a Robust Portfolio:
Initiate Personal Projects: Embark on personal data science projects to display your skills and ingenuity. This might involve analyzing datasets, constructing predictive models, or crafting visualizations.
Maintain a GitHub Repository: Keep a GitHub repository to share your code and projects with potential employers. A well-maintained portfolio exhibits your expertise and enthusiasm for data science.
4. Networking:
Participate in Conferences and Meetups: Networking with field professionals can pave the way for collaboration or job opportunities. Attend industry events, conferences, and local meetups centered around data science.
Join Online Communities: Become a part of online communities like LinkedIn groups, Reddit forums (e.g., r/datascience), or Slack channels focused on data science to connect with peers.
5. Job Hunting Strategies:
Browse Job Boards: Check out job boards specific to data science roles such as Indeed, Glassdoor, or DataJobs.com. Customize your resume and cover letter to emphasize relevant skills and experiences.
Use Career Services: Take advantage of the career services provided by your school or seek advice from professionals in the field regarding job hunting strategies.
By diligently following these steps and continuously learning and honing your skills, you can successfully navigate your way to a fulfilling data science career.
Top 3 Credible Sources Used:
Harvard Business Review: Offers insights into the latest trends and best practices in data science, providing valuable information on career growth strategies.
Towards Data Science: A digital platform that shares articles on a range of data science topics, including guides on charting a career path in the field and practical advice for budding data scientists.
DataCamp: An interactive educational platform that provides courses on programming languages like Python and R, as well as specialized training modules on data analysis and machine learning techniques crucial for budding data scientists.
These sources were key in providing accurate information on charting a data science career path based on industry standards and best practices.
God Bless You,
James.
ME:-
If for instance you have nutrition and dietetics and computing, using data you can make software that educates people about what the Standard Reference database contains. Levels of nutrients that can ameliorate sicknesses. An example is zinc supporting immune function.
THE AI:-
Charting a Path Towards a Data Science Career
Embarking on a data science career, particularly if you have a computer science background, involves several steps to boost your skills and improve your chances of success. Here's a detailed roadmap to navigate your way into a data science career:
1. Education and Skill Enhancement:
Pursue Relevant Education: Start by obtaining a degree in a related field such as computer science, statistics, mathematics, or data science. These areas form the bedrock of a data science career.
Enroll in Specialized Courses and Certifications: Consider signing up for specialized data science courses or earning certifications. Online platforms like Coursera, edX, and Udacity offer a variety of data science courses.
Hone Programming Skills: Mastery of programming languages like Python, R, SQL, and Java is vital for data science roles. Regular coding practice will solidify your skills.
Understand Statistics: Grasping statistical concepts and techniques is key to effectively analyzing and interpreting data.
2. Accumulate Practical Experience:
Seek Internships and Projects: Pursue internships or projects that enable you to use your knowledge in real-life situations. Practical experience will not only augment your skills but also make you more appealing to potential employers.
Join Kaggle Competitions: Engaging in Kaggle competitions can offer valuable experience in tackling intricate data problems and working with actual datasets.
3. Construct a Robust Portfolio:
Initiate Personal Projects: Embark on personal data science projects to display your skills and ingenuity. This might involve analyzing datasets, constructing predictive models, or crafting visualizations.
Maintain a GitHub Repository: Keep a GitHub repository to share your code and projects with potential employers. A well-maintained portfolio exhibits your expertise and enthusiasm for data science.
4. Networking:
Participate in Conferences and Meetups: Networking with field professionals can pave the way for collaboration or job opportunities. Attend industry events, conferences, and local meetups centered around data science.
Join Online Communities: Become a part of online communities like LinkedIn groups, Reddit forums (e.g., r/datascience), or Slack channels focused on data science to connect with peers.
5. Job Hunting Strategies:
Browse Job Boards: Check out job boards specific to data science roles such as Indeed, Glassdoor, or DataJobs.com. Customize your resume and cover letter to emphasize relevant skills and experiences.
Use Career Services: Take advantage of the career services provided by your school or seek advice from professionals in the field regarding job hunting strategies.
By diligently following these steps and continuously learning and honing your skills, you can successfully navigate your way to a fulfilling data science career.
Top 3 Credible Sources Used:
Harvard Business Review: Offers insights into the latest trends and best practices in data science, providing valuable information on career growth strategies.
Towards Data Science: A digital platform that shares articles on a range of data science topics, including guides on charting a career path in the field and practical advice for budding data scientists.
DataCamp: An interactive educational platform that provides courses on programming languages like Python and R, as well as specialized training modules on data analysis and machine learning techniques crucial for budding data scientists.
These sources were key in providing accurate information on charting a data science career path based on industry standards and best practices.
God Bless You,
James.
Updated
Sean’s Answer
Data science is like the friendly neighbor of computer science. They share many similarities, and having a robust collection of projects under your belt is a surefire way to succeed. Here are some friendly tips to guide you on this exciting journey:
1. Build a Strong Base: Make sure you're well-versed in programming languages like Python, R, and have a good grasp of statistics and mathematics.
2. Get to Know Data Science Tools: Get comfortable with data science tools and libraries like Pandas, NumPy, Matplotlib, Scikit-learn, TensorFlow, and PyTorch.
3. Online Learning and Certifications: Sign up for online courses on sites like Coursera and Udemy. Look out for certifications in data science and machine learning.
4. Join Competitions: Participate in data science competitions on platforms like Kaggle to solve real-life problems and sharpen your skills.
5. Showcase Your Work: Set up a GitHub repository to display your projects. This is a great way to show potential employers what you're capable of.
6. Make Connections: Go to data science meetups, join professional groups, and link up with industry professionals on LinkedIn.
7. Get Hands-On Experience: Seek out internships to gain practical experience. Keep an eye out for opportunities in tech companies, startups, or research labs.
Here are some project ideas for data science:
1. Predictive Analytics: Construct a predictive model for things like stock prices, sales forecasts, or customer churn using machine learning algorithms.
2. Natural Language Processing (NLP): Make a sentiment analysis tool, chatbot, or text summarization system.
3. Data Visualization: Create dynamic dashboards with tools like Tableau or Plotly to make complex datasets easier to understand.
4. Recommendation Systems: Design a recommendation engine like the ones used by Netflix or Amazon.
5. Image Recognition: Build a convolutional neural network (CNN) for tasks like image classification or object detection.
6. Health Informatics: Analyze medical data to predict things like disease outbreaks, patient readmission rates, or treatment outcomes.
7. Social Media Analytics: Examine Twitter data to track trends, sentiments, or user engagement.
8. Time Series Analysis: Tackle projects involving time series data, such as predicting weather patterns or energy usage.
I hope these tips help. Best of luck on your data science journey!
1. Build a Strong Base: Make sure you're well-versed in programming languages like Python, R, and have a good grasp of statistics and mathematics.
2. Get to Know Data Science Tools: Get comfortable with data science tools and libraries like Pandas, NumPy, Matplotlib, Scikit-learn, TensorFlow, and PyTorch.
3. Online Learning and Certifications: Sign up for online courses on sites like Coursera and Udemy. Look out for certifications in data science and machine learning.
4. Join Competitions: Participate in data science competitions on platforms like Kaggle to solve real-life problems and sharpen your skills.
5. Showcase Your Work: Set up a GitHub repository to display your projects. This is a great way to show potential employers what you're capable of.
6. Make Connections: Go to data science meetups, join professional groups, and link up with industry professionals on LinkedIn.
7. Get Hands-On Experience: Seek out internships to gain practical experience. Keep an eye out for opportunities in tech companies, startups, or research labs.
Here are some project ideas for data science:
1. Predictive Analytics: Construct a predictive model for things like stock prices, sales forecasts, or customer churn using machine learning algorithms.
2. Natural Language Processing (NLP): Make a sentiment analysis tool, chatbot, or text summarization system.
3. Data Visualization: Create dynamic dashboards with tools like Tableau or Plotly to make complex datasets easier to understand.
4. Recommendation Systems: Design a recommendation engine like the ones used by Netflix or Amazon.
5. Image Recognition: Build a convolutional neural network (CNN) for tasks like image classification or object detection.
6. Health Informatics: Analyze medical data to predict things like disease outbreaks, patient readmission rates, or treatment outcomes.
7. Social Media Analytics: Examine Twitter data to track trends, sentiments, or user engagement.
8. Time Series Analysis: Tackle projects involving time series data, such as predicting weather patterns or energy usage.
I hope these tips help. Best of luck on your data science journey!
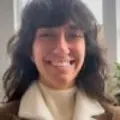
Constanza Moyano-Schmidt
Senior Product Designer / Conversational AI Designer / Design Mentor
2
Answers
South Lake Tahoe, California
Updated
Constanza’s Answer
Hello Melissa,
Embarking on a journey towards a data science career can be an exciting adventure, filled with numerous stages that aren't always straightforward. It requires an immense level of commitment, tenacity, inquisitiveness, and a love for untangling intricate issues – these are the essential ingredients to thrive in this arena.
By steadfastly adhering to these steps and consistently polishing your abilities, you're on the right path to carve out a fulfilling career in the realm of data science.
Start with a strong foundation in mathematics, statistics, and computer science. Many data scientists hold degrees in fields such as mathematics, statistics, computer science, or related disciplines. Consider pursuing formal education through bachelor's or master's degree programs in data science, statistics, computer science, or a related field. Additionally, there are numerous online courses, bootcamps, and self-study resources available for learning data science skills.
Develop proficiency in programming languages commonly used in data science, such as Python or R. Learn how to manipulate and analyze data using libraries and tools like pandas, NumPy, and scikit-learn in Python, or tidyverse packages in R. Familiarize yourself with data visualization tools like Matplotlib, Seaborn, ggplot2, and Tableau. Additionally, acquire knowledge of databases and SQL for data manipulation and retrieval.
Build a Portfolio: Showcase your skills and expertise by working on data science projects. Start with small projects and gradually tackle more complex problems. Participate in online competitions like those hosted on Kaggle, where you can solve real-world data science problems and collaborate with other data enthusiasts. Your portfolio should demonstrate your ability to gather, clean, analyze, and visualize data to derive meaningful insights.
Understand the industry or domain you are interested in working in. Whether it's healthcare, finance, e-commerce, or any other sector, having domain knowledge will help you better understand the data and make more informed decisions. Consider taking courses or reading books related to your chosen field to deepen your understanding.
Attend data science meetups, conferences, and networking events to connect with professionals in the field. Engage with online communities, forums, and social media platforms where data scientists share knowledge, insights, and job opportunities. Networking can help you learn from others, gain valuable advice, and potentially find job opportunities.
Embarking on a journey towards a data science career can be an exciting adventure, filled with numerous stages that aren't always straightforward. It requires an immense level of commitment, tenacity, inquisitiveness, and a love for untangling intricate issues – these are the essential ingredients to thrive in this arena.
By steadfastly adhering to these steps and consistently polishing your abilities, you're on the right path to carve out a fulfilling career in the realm of data science.
Constanza recommends the following next steps:
Updated
Jaquan’s Answer
Besides jumping right into it by applying for opportunities pursuing a career in data science involves a combination of acquiring academic knowledge, developing practical skills, and gaining relevant work experience. Here are some steps you can follow:
1. Obtain Relevant Education
While not always a strict requirement, having a degree can be beneficial in the data science field. A degree in fields like computer science, statistics, mathematics, or data science can provide a strong foundation. Many universities also offer postgraduate programs specifically in data science.
2. Develop Your Mathematical and Statistical Skills
Data science requires a strong grasp of mathematics and statistics, as they are the basis for many data science techniques and algorithms. You'll need to understand areas like linear algebra, calculus, probability, and statistical analysis.
3. Learn Programming Languages
Python and R are the most commonly used programming languages in data science. SQL is also important, as it's used for database management and data extraction.
4. Get Familiar with Data Science Tools and Libraries
Familiarize yourself with tools commonly used in data science, such as Jupyter notebooks, Tableau, and Apache Hadoop. Learn to use libraries like Pandas, NumPy, Matplotlib, and Scikit-learn for data analysis and manipulation in Python.
5. Understand Machine Learning
Machine learning is a key component of data science. Gain a solid understanding of machine learning algorithms, and how and when to use them.
6. Work on Projects
Hands-on experience is crucial. Work on personal projects, participate in data science competitions on platforms like Kaggle, or contribute to open-source projects. This allows you to apply what you've learned and gain practical skills.
7. Gain Real-World Experience
Internships or entry-level jobs in data science or related fields can provide invaluable experience. They also help you understand how data science principles are applied in a professional setting.
8. Keep Learning
The field of data science is constantly evolving, so continuous learning is key. Stay updated on the latest trends and advancements, and continually work on improving and expanding your skills.
9. Build a Portfolio
A portfolio showcasing your projects and skills can be a powerful tool when applying for jobs. It gives potential employers a tangible demonstration of what you can do.
Remember, breaking into data science can take time and persistence, but the demand for data scientists is high, and it's a field with great potential for growth and advancement.
1. Obtain Relevant Education
While not always a strict requirement, having a degree can be beneficial in the data science field. A degree in fields like computer science, statistics, mathematics, or data science can provide a strong foundation. Many universities also offer postgraduate programs specifically in data science.
2. Develop Your Mathematical and Statistical Skills
Data science requires a strong grasp of mathematics and statistics, as they are the basis for many data science techniques and algorithms. You'll need to understand areas like linear algebra, calculus, probability, and statistical analysis.
3. Learn Programming Languages
Python and R are the most commonly used programming languages in data science. SQL is also important, as it's used for database management and data extraction.
4. Get Familiar with Data Science Tools and Libraries
Familiarize yourself with tools commonly used in data science, such as Jupyter notebooks, Tableau, and Apache Hadoop. Learn to use libraries like Pandas, NumPy, Matplotlib, and Scikit-learn for data analysis and manipulation in Python.
5. Understand Machine Learning
Machine learning is a key component of data science. Gain a solid understanding of machine learning algorithms, and how and when to use them.
6. Work on Projects
Hands-on experience is crucial. Work on personal projects, participate in data science competitions on platforms like Kaggle, or contribute to open-source projects. This allows you to apply what you've learned and gain practical skills.
7. Gain Real-World Experience
Internships or entry-level jobs in data science or related fields can provide invaluable experience. They also help you understand how data science principles are applied in a professional setting.
8. Keep Learning
The field of data science is constantly evolving, so continuous learning is key. Stay updated on the latest trends and advancements, and continually work on improving and expanding your skills.
9. Build a Portfolio
A portfolio showcasing your projects and skills can be a powerful tool when applying for jobs. It gives potential employers a tangible demonstration of what you can do.
Remember, breaking into data science can take time and persistence, but the demand for data scientists is high, and it's a field with great potential for growth and advancement.
Updated
Nick’s Answer
This is an excellent, direct question! I work more on data analysis than on data science; however, many of the tools that I look at cross over with the data science field. My first thought would be is to learn Python and R. There are many leading tools for data science these days, but most employers tend to look for these two languages. They do pretty much the same things, but R is more catered towards statistics. I personally use a lot of Python in my work!
My next thought would be to learn about what data you're interested in learning more about! I work primarily with accounting data, as the size of accounting data by company increases substantially every year, it's important to have data science tools to break down and summarize this data. I love accounting and I have a strong background in accounting, so it's actually very fun for me to break down this information and learn more about it.
So ask yourself, what do you want to learn about? It would be helpful if you not only know you want to learn data science, but to specialize your understanding to datasets that you are passionate about! Then, you can start to apply descriptive, predictive, and prescriptive data science techniques on those datasets and add value to companies in big ways. Data science is a really sought after position right now; you're in a good place to be interested in this field!
My next thought would be to learn about what data you're interested in learning more about! I work primarily with accounting data, as the size of accounting data by company increases substantially every year, it's important to have data science tools to break down and summarize this data. I love accounting and I have a strong background in accounting, so it's actually very fun for me to break down this information and learn more about it.
So ask yourself, what do you want to learn about? It would be helpful if you not only know you want to learn data science, but to specialize your understanding to datasets that you are passionate about! Then, you can start to apply descriptive, predictive, and prescriptive data science techniques on those datasets and add value to companies in big ways. Data science is a really sought after position right now; you're in a good place to be interested in this field!